Data Foundation Experts
Building Robust Data Infrastructure for Swift Insights and ML Excellence
Empowering Data-Driven Success
In the pursuit of swift insights from data and seamless utilization of ML models, the cornerstone is laying down a robust data foundation. This entails structuring data in a manner that facilitates rapid and dependable access, essential for both analysis and ML model development.
Our proficiency extends to devising meticulous data design processes, ensuring a repeatable framework for acquiring insights and building ML models.
Our Expertise
We specialize in steering IT system integrators towards establishing optimal data structures, implementing efficient data reconciliation methods, upholding rigorous data governance practices, and maintaining adept database management protocols.
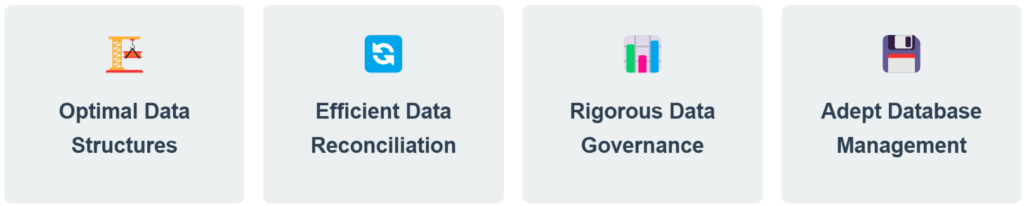
Our Objective
Our objective is to fortify the underlying data infrastructure, rendering it:
- Reliable: Ensuring data integrity and consistency
- User-friendly: Facilitating easy access and interpretation
- Conducive to streamlined processing: Optimizing for speed and efficiency
By focusing on these key aspects, we empower organizations to harness the full potential of their data, driving informed decision-making and fostering innovation.
Nitty-Gritty of Data Strategy
📊 Data Strategy for Analytics:
🎯 Model Development Perspective:
-
- A clear strategy is imperative for model development, necessitating the ability to maintain account-level and monthly data.
- Procedures should be in place to generate account-level data spanning multiple months, incorporating defined methodologies such as aggregations (e.g., last, sum, average, max, min).
📊 Business Intelligence (BI) Perspective:
-
- Data must be structured in a cubical format, summarized at fixed dimension levels for most of the dashboards.
- Well-defined functions should be established to utilize cubical data accurately across various levels.
- The strategy should account for varying BI requirements, from account-level to transaction-level records, prioritizing the most frequent to less frequent BI needs.
🚀 Campaign Management Perspective:
-
- Both account-level and transaction-level data are essential for campaign management.
- Data storage considerations include month-end and cycle-level data.
🔧 Common Strategies Across All Perspectives:
-
- Define storage strategies for recent, historical, and archival data.
- Establish protocols for regular automated data quality checks.
- Implement triggers to preemptively address errors or issues in subsequent processes.
- Design a careful hierarchy for data granularity, ensuring seamless reconciliation and access across customer, account, and card levels in scenarios like credit card business.
This comprehensive approach ensures a cohesive data strategy that caters to the specific needs of model development, business intelligence, and campaign management, while maintaining consistency and reliability across the board.